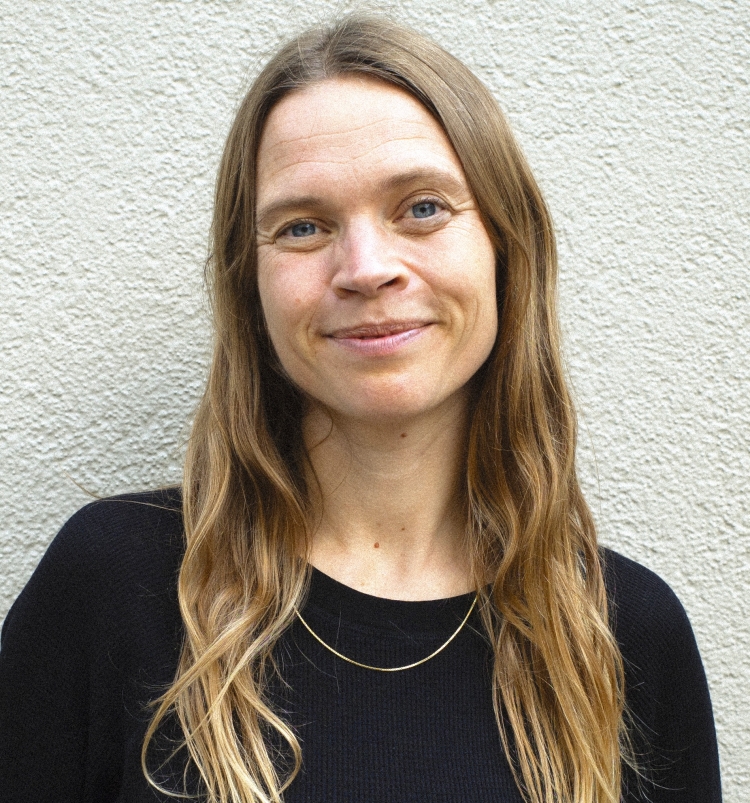
The 2025 Young Experimental Physicist Prize is awarded jointly to Thea Klæboe Årrestad (CMS) and Laura Zani for their remarkable and complementary contributions to experimental particle physics. The prize has been awarded by the High Energy and Particle Physics Division of the European Physics Society.
Thea Årrestad is recognised for her groundbreaking work in integrating modern machine learning techniques into particle physics, most notably in the domain of real-time data processing and event selection. Through her pioneering contributions, she has developed a new approach on how collider experiments handle the immense data flow produced at the LHC.
This work has been instrumental in the creation of the first anomaly-detection-based trigger in CMS, marking a paradigm shift for particle physics experiments towards real-time AI-driven decision-making. It uses machine learning strategies that train a neural network to characterise how typical a given event is. This is then used as a metric to identify rare events that could come from some unspecified new physics signal.
Thanks to Thea’s effort, CMS is leading the field in the use of deep neural networks in the Level 1 trigger and anomaly detection algorithms. Thea has worked to build a thriving community around deep learning in physics, working tirelessly to train a new generation of deep learning experts and has demonstrated her leadership in building open-source tools such as hls4ml and QKeras. Her collaborations with external partners like Google and Volvo’s Zenseact have led to breakthroughs in neural network compression, of which the CMS collaboration, particle physics, and society in general are reaping the benefits.
Congratulations Thea!
ABOVE: Thea in front of one of the CMS "test crates", where she and the team implemented and tested their first ML-based Level-1 trigger anomaly detection called AXOL1TL.
Further Reading:
The EPS Young Experimental Physicist Prize
The first proof-of-principle of anomaly detection on FPGAs for triggering, published in Nature
First data collected with AXOL1TL
Anomaly detection analysis (currently under review for publication 28/04/25)
Review paper on anomaly detection in HEP written for Science Direct
hls4ml - Python package for machine learning inference in FPGAs
QKeras - quantization extention to Keras, a high-level API to build and train deep learning models